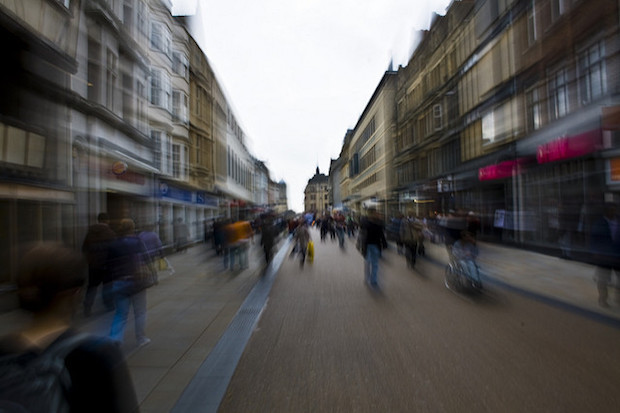
Many local governments have reams of data (both hard data and soft data) on local inhabitants and local businesses. Image: Chris Dawkins (Flickr CC BY-NC-ND 2.0).
The concept of Big Data has become very popular over the last decade, with many large technology companies successfully building their business models around its exploitation. The UK’s public sector has tried to follow suit, with local governments in particular trying to introduce new models of service delivery based on the routine extraction of information from their own big data. These attempts have been hailed as the beginning of a new era for the public sector, with some commentators suggesting that it could help local governments transition toward a model of service delivery where the quantity and quality of commissioned services is underpinned by data intelligence on users and their current and future needs. In their Policy & Internet article “Data Intelligence for Local Government? Assessing the Benefits and Barriers to Use of Big Data in the Public Sector”, Fola Malomo and Vania Sena examine the extent to which local governments in the UK are indeed using intelligence from big data, in light of the structural barriers they face when trying to exploit it. Their analysis suggests that the ambitions around the development of big data capabilities in local government are not reflected in actual use. Indeed, these methods have mostly been employed to develop new digital channels for service delivery, and even if the financial benefits of these initiatives are documented, very little is known about the benefits generated by them for the local communities. While this is slowly changing as councils start to develop their big data capability, the overall impression gained from even a cursory overview is that the full potential of big data is yet to be exploited. We caught up with the authors to discuss their findings: Ed.: So what actually is “the full potential” that local government is supposed to be aiming for? What exactly is the promise of “big data” in this context? Fola / Vania: Local governments seek to improve service delivery…