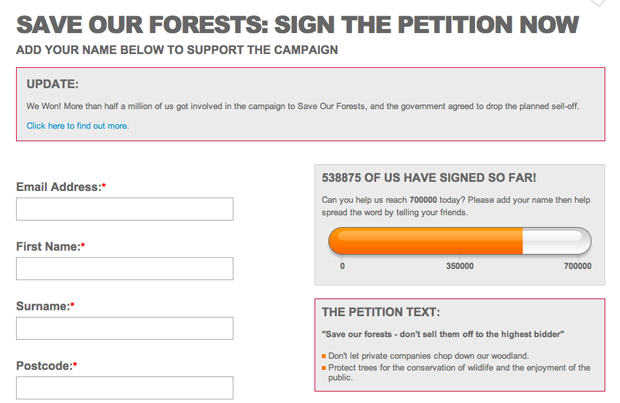
Following a furious public backlash in 2011, the UK government abandoned plans to sell off 258,000 hectares of state-owned woodland. The public forest campaign by 38 Degrees gathered over half a million signatures.
How do we define political participation? What does it mean to say an action is ‘political’? Is an action only ‘political’ if it takes place in the mainstream political arena; involving government, politicians or voting? Or is political participation something that we find in the most unassuming of places, in sports, home and work? This question, ‘what is politics’ is one that political scientists seem to have a lot of trouble dealing with, and with good reason. If we use an arena definition of politics, then we marginalise the politics of the everyday; the forms of participation and expression that develop between the cracks, through need and ingenuity. However, if we broaden our approach as so to adopt what is usually termed a process definition, then everything can become political. The problem here is that saying that everything is political is akin to saying nothing is political, and that doesn’t help anyone. Over the years, this debate has plodded steadily along, with scholars on both ends of the spectrum fighting furiously to establish a working understanding. Then, the Internet came along and drew up new battle lines. The Internet is at its best when it provides a home for the disenfranchised, an environment where like-minded individuals can wipe free the dust of societal disassociation and connect and share content. However, the Internet brought with it a shift in power, particularly in how individuals conceptualised society and their role within it. The Internet, in addition to this role, provided a plethora of new and customisable modes of political participation. From the onset, a lot of these new forms of engagement were extensions of existing forms, broadening the everyday citizen’s participatory repertoire. There was a move from voting to e-voting, petitions to e-petitions, face-to-face communities to online communities; the Internet took what was already there and streamlined it, removing those pesky elements of time, space and identity. Yet, as the Internet continues…