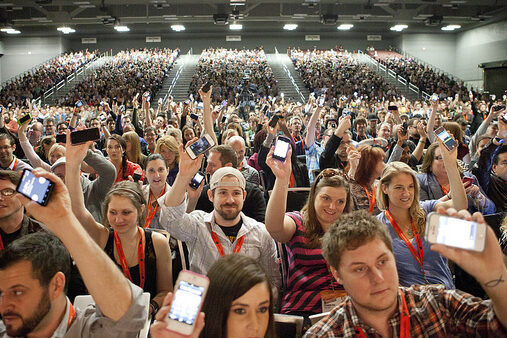
What is the difference between popularity and reputation on the process of social influence? Image: kris krüg (Flickr CC BY-NC-ND 2.0).
A significant part of political deliberation now takes place on online forums and social networking sites, leading to the idea that collective action might be evolving into “connective action”. The new level of connectivity (particularly of social media) raises important questions about its role in the political process. But understanding important phenomena, such as social influence, social forces, and digital divides, requires analysis of very large social systems, which traditionally has been a challenging task in the social sciences. In their Policy & Internet article “Understanding Popularity, Reputation, and Social Influence in the Twitter Society”, David Garcia, Pavlin Mavrodiev, Daniele Casati, and Frank Schweitzer examine popularity, reputation, and social influence on Twitter using network information on more than 40 million users. They integrate measurements of popularity, reputation, and social influence to evaluate what keeps users active, what makes them more popular, and what determines their influence in the network. Popularity in the Twitter social network is often quantified as the number of followers of a user. That implies that it doesn’t matter why some user follows you, or how important she is, your popularity only measures the size of your audience. Reputation, on the other hand, is a more complicated concept associated with centrality. Being followed by a highly reputed user has a stronger effect on one’s reputation than being followed by someone with low reputation. Thus, the simple number of followers does not capture the recursive nature of reputation. In their article, the authors examine the difference between popularity and reputation on the process of social influence. They find that there is a range of values in which the risk of a user becoming inactive grows with popularity and reputation. Popularity in Twitter resembles a proportional growth process that is faster in its strongly connected component, and that can be accelerated by reputation when users are already popular. They find that social influence on Twitter is mainly related to…