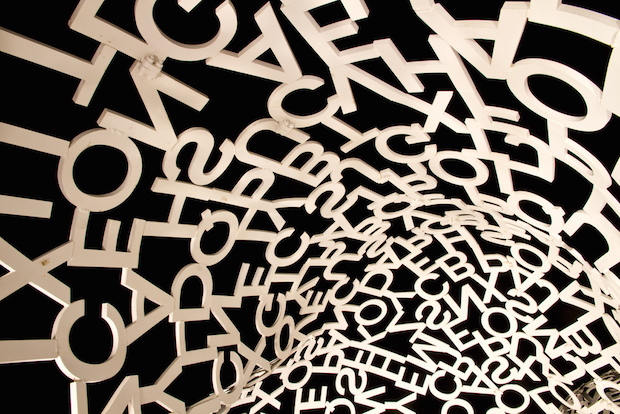
Wikipedia uses editing bots to clean articles: but what happens when their interactions go bad? Image of "Nomade", a sculpture in downtown Des Moines by Jason Mrachina (Flickr CC BY-NC-ND 2.0).
Recent years have seen a huge increase in the number of bots online—including search engine Web crawlers, online customer service chat bots, social media spambots, and content-editing bots in online collaborative communities like Wikipedia. (Bots are important contributors to Wikipedia, completing about 15% of all Wikipedia edits in 2014 overall, and more than 50% in certain language editions.) While the online world has turned into an ecosystem of bots (by which we mean computer scripts that automatically handle repetitive and mundane tasks), our knowledge of how these automated agents interact with each other is rather poor. But being automata without capacity for emotions, meaning-making, creativity, or sociality, we might expect bot interactions to be relatively predictable and uneventful. In their PLOS ONE article “Even good bots fight: The case of Wikipedia”, Milena Tsvetkova, Ruth García-Gavilanes, Luciano Floridi, and Taha Yasseri analyse the interactions between bots that edit articles on Wikipedia. They track the extent to which bots undid each other’s edits over the period 2001–2010, model how pairs of bots interact over time, and identify different types of interaction outcomes. Although Wikipedia bots are intended to support the encyclopaedia—identifying and undoing vandalism, enforcing bans, checking spelling, creating inter-language links, importing content automatically, mining data, identifying copyright violations, greeting newcomers, etc.—the authors find they often undid each other’s edits, with these sterile “fights” sometimes continuing for years. They suggest that even relatively “dumb” bots may give rise to complex interactions, carrying important implications for Artificial Intelligence research. Understanding these bot-bot interactions will be crucial for managing social media, providing adequate cyber-security, and designing autonomous vehicles (that don’t crash). We caught up with Taha Yasseri and Luciano Floridi to discuss the implications of the findings: Ed.: Is there any particular difference between the way individual bots interact (and maybe get bogged down in conflict), and lines of vast and complex code interacting badly, or having unforeseen results (e.g. flash-crashes in automated trading):…