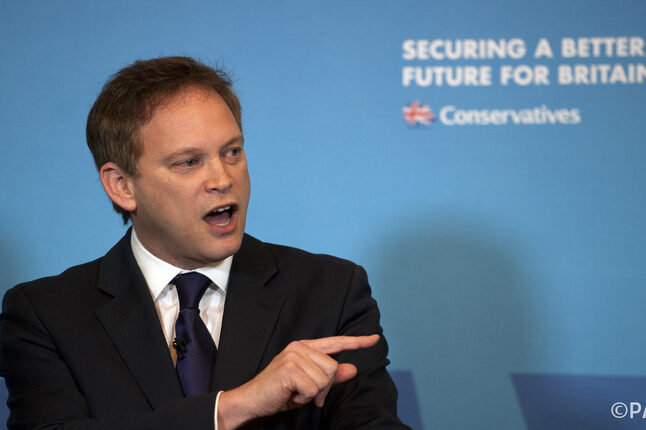
Conservative Party Chairman Grant Shapps gives a speech on free trade at the Institute of Directors in London.
Reposted from The Conversation. Wikipedia has become one of the most highly linked-to websites on the internet, with countless others using it as a reference. But it can be edited by anyone, and this has led to occasions where errors have been widely repeated—or where facts have been distorted to fit an agenda. The chairman of the UK’s Conservative Party, Grant Shapps, has been accused of editing Wikipedia pages related to him and his rivals within the party. The Guardian newspaper claims Wikipedia administrators blocked an account on suspicions that it was being used by Shapps, or someone in his employ. Wikipedia accounts are anonymous, so what is the support for these claims? Is it a case of fair cop or, as Shapps says in his defence, a smear campaign in the run-up to the election? Edits examined This isn’t the first time The Guardian has directed similar accusations against Shapps around edits to Wikipedia, with similar claims emerging in September 2012. The investigation examines a list of edits by three Wikipedia user accounts: Hackneymarsh, Historyset, and Contribsx, and several other edits from users without accounts, recorded only as their IP addresses—which the article claimed to be “linked” to Shapps. The Hackneymarsh account made 12 edits in a short period in May 2010. The Historyset account made five edits in a similar period. All the edits recorded by IP addresses date to between 2008 and 2010. Most recently, the Contribsx account has been active from August 2013 to April 2015. First of all, it is technically impossible to conclusively link any of those accounts or IP addresses to a real person. Of course you can speculate—and in this case it’s clear that these accounts seem to demonstrate great sympathy with Shapps based on the edits they’ve made. But no further information about the three usernames can be made public by the Wikimedia Foundation, as per its privacy policies. However, the case is different for the IP addresses. Using GeoIP or…