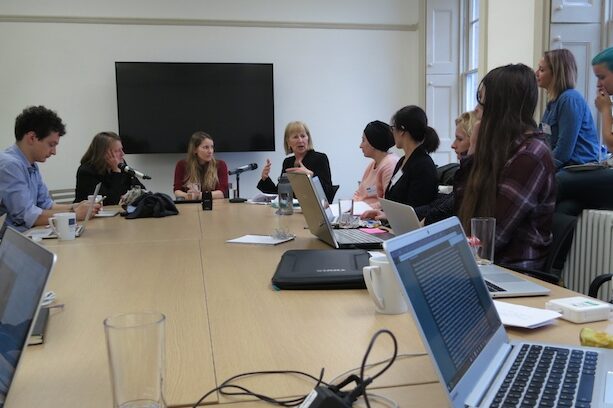
Conversation between Laura Bates, Judy Wajcman (speaking) and Helen Margetts, at the Everyday Sexism Datahack, organised by the OII to encourage creative engagement with the textual data gathered by the Everyday Sexism project.
To encourage new ways of thinking about the problem of sexism in daily life, the OII’s recent Everyday Sexism Datahack brought together twenty people from a range of disciplinary backgrounds to analyse the written accounts of sexism and harassment gathered by the Everyday Sexism project. Founded by Laura Bates in 2012, Everyday Sexism has gathered more than 120,000 accounts submitted by members of the public. A research team at the OII has already been analysing the content, and provided cleaned data to the datahack participants that could be analysed through qualitative and quantitative methods. Following an introduction to the project by Laura Bates, an outline of the dataset by Taha Yasseri, and a speed-networking session led by Kathryn Eccles we fell into two teams to work with the data. Our own group wanted to examine the question of how people interact with the threat of public space. We were also interested in how public space is divided between online and offline, and the social perception of being online versus offline. We wanted to explore what sorts of reactions people might have to examples of assault, or strategies or things they might do in response to something happening to them—and how they might differ online and offline. We spent the first hour collecting keywords that might indicate reactions to either online or offline harassment, including identifying a perceived threat and coping with it. We then searched the raw data for responses like “I tried to ignore it,” “I felt safe/unsafe,” “I identified a risk,” “I was feeling worried, feeling anxious or nervous”; and also looked at online versus offline actions. So for online action we were looking for specific platforms being named, and people saying things like “comment, response, delete, remove” in relation to social media posts. For offline we were looking for things like “I carried a [specific item]” or “I hid or avoided certain areas“ or “I walked faster”…