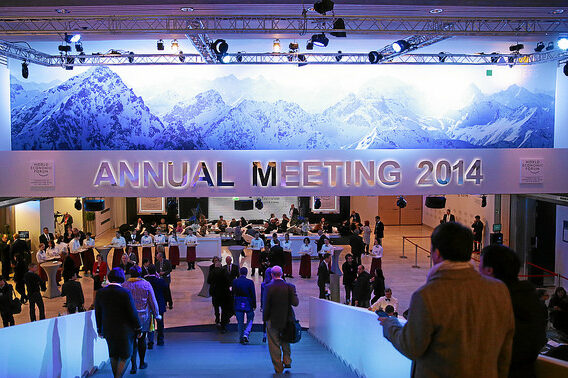
The World Economic Forum engages business, political, academic and other leaders of society to shape global, regional and industry agendas. Image by World Economic Forum.
Last week, I was at the World Economic Forum in Davos, the first time that the Oxford Internet Institute has been represented there. Being closeted in a Swiss ski resort with 2,500 of the great, the good and the super-rich provided me with a good chance to see what the global elite are thinking about technological change and its role in ‘The Reshaping of the World: Consequences for Society, Politics and Business’, the stated focus of the WEF Annual Meeting in 2014. What follows are those impressions that relate to public policy and the internet, and reflect only my own experience there. Outside the official programme there are whole hierarchies of breakfasts, lunches, dinners and other events, most of which a newcomer to Davos finds it difficult to discover and some of which require one to be at least a president of a small to medium-sized state—or Matt Damon. There was much talk of hyperconnectivity, spirals of innovation, S-curves and exponential growth of technological diffusion, digitalisation and disruption. As you might expect, the pace of these was emphasised most by those participants from the technology industry. The future of work in the face of leaps forward in robotics was a key theme, drawing on the new book by Eric Brynjolfsson and Andrew McAfee, The Second Machine Age: Work, Progress and Prosperity in a Time of Brilliant Technologies, which is just out in the US. There were several sessions on digital health and the eventual fruition of decades of pilots in telehealth (a banned term now, apparently), as applications based on mobile technologies start to be used more widely. Indeed, all delegates were presented with a ‘Jawbone’ bracelet which tracks the wearer’s exercise and sleep patterns (7,801 steps so far today). And of course there was much talk about the possibilities afforded by big data, if not quite as much as I expected. The University of Oxford was represented in an…