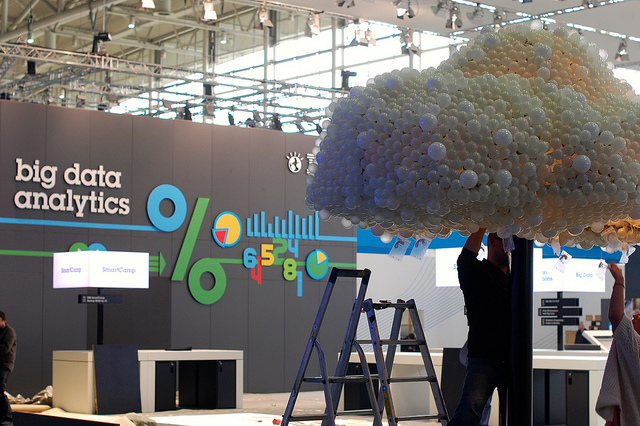
There is a lot of excitement about 'big data', but the potential for innovative work on social and cultural topics far outstrips current data collection and analysis techniques. Image by IBM Deutschland.
Using anything digital always creates a trace. The more digital ‘things’ we interact with, from our smart phones to our programmable coffee pots, the more traces we create. When collected together these traces become big data. These collections of traces can become so large that they are difficult to store, access and analyse with today’s hardware and software. But as a social scientist I’m interested in how this kind of information might be able to illuminate something new about societies, communities, and how we interact with one another, rather than engineering challenges.
Social scientists are just beginning to grapple with the technical, ethical, and methodological challenges that stand in the way of this promised enlightenment. Most of us are not trained to write database queries or regular expressions, or even to communicate effectively with those who are trained. Ethical questions arise with informed consent when new analytics are created. Even a data scientist could not know the full implications of consenting to data collection that may be analysed with currently unknown techniques. Furthermore, social scientists tend to specialise in a particular type of data and analysis, surveys or experiments and inferential statistics, interviews and discourse analysis, participant observation and ethnomethodology, and so on. Collaborating across these lines is often difficult, particularly between quantitative and qualitative approaches. Researchers in these areas tend to ask different questions and accept different kinds of answers as valid.
Yet trace data does not fit into the quantitative/qualitative binary. The trace of a tweet includes textual information, often with links or images and metadata about who sent it, when and sometimes where they were. The traces of web browsing are also largely textual with some audio/visual elements. The quantity of these textual traces often necessitates some kind of initial quantitative filtering, but it doesn’t determine the questions or approach.
The challenges are important to understand and address because the promise of new insight into social life is real. Large-scale patterns become possible to detect, for example according to one study of mobile phone location data one’s future location is 93% predictable (Song, Qu, Blum & Barabási, 2010), despite great variation in the individual patterns. This new finding opens up further possibilities for comparison and understanding the context of these patterns. Are locations more or less predictable among people with different socio-economic circumstances? What are the key differences between the most and least predictable?
Computational social science is often associated with large-scale studies of anonymised users such as the phone location study mentioned above, or participation traces of those who contribute to online discussions. Studies that focus on limited information about a large number of people are only one type, which I call horizontal trace data. Other studies that work in collaboration with informed participants can add context and depth by asking for multiple forms of trace data and involving participants in interpreting them—what I call the vertical trace data approach.
In my doctoral dissertation I took the vertical approach to examining political information gathering during an election, gathering participants’ web browsing data with their informed consent and interviewing them in person about the context (Menchen-Trevino 2012). I found that access to websites with political information was associated with self-reported political interest, but access to election-specific pages was not. The most active election-specific browsing came from those who were undecided on election day, while many of those with high political interest had already decided whom to vote for before the election began. This is just one example of how digging futher into such data can reveal that what is true for larger categories (political information in general) may not be true, and in fact can be misleading for smaller domains (election-specific browsing). Vertical trace data collection is difficult, but it should be an important component of the project of computational social science.
Read the full article: Menchen-Trevino, E. (2013) Collecting vertical trace data: Big possibilities and big challenges for multi-method research. Policy and Internet 5 (3) 328-339.
References
Menchen-Trevino, E. (2013) Collecting vertical trace data: Big possibilities and big challenges for multi-method research. Policy and Internet 5 (3) 328-339.
Menchen-Trevino, E. (2012) Partisans and Dropouts?: News Filtering in the Contemporary Media Environment. Northwestern University, Evanston, Illinois.
Song, C., Qu, Z., Blumm, N., & Barabasi, A.-L. (2010) Limits of Predictability in Human Mobility. Science 327 (5968) 1018–1021.
Erica Menchen-Trevino is an Assistant Professor at Erasmus University Rotterdam in the Media & Communication department. She researches and teaches on topics of political communication and new media, as well as research methods (quantitative, qualitative and mixed).