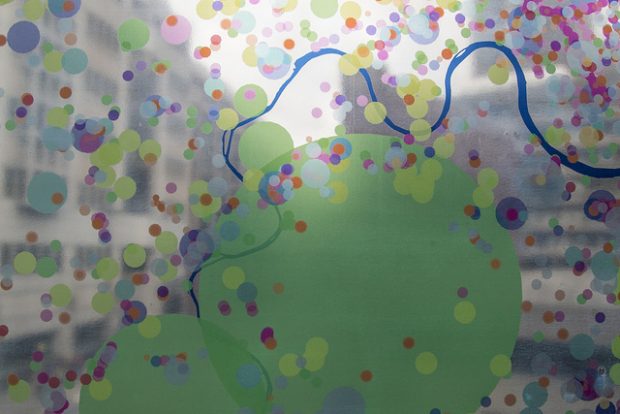
Advocates hope that opening government data will increase government transparency, catalyse economic growth, address social and environmental challenges. Image by the UK’s Open Data Institute.
Community-based approaches are widely employed in programmes that monitor and promote socioeconomic development. And building the “capacity” of a community—i.e. the ability of people to act individually or collectively to benefit the community—is key to these approaches. The various definitions of community capacity all agree that it comprises a number of dimensions—including opportunities and skills development, resource mobilisation, leadership, participatory decision making, etc.—all of which can be measured in order to understand and monitor the implementation of community-based policy. However, measuring these dimensions (typically using surveys) is time consuming and expensive, and the absence of such measurements is reflected in a greater focus in the literature on describing the process of community capacity building, rather than on describing how it’s actually measured. A cheaper way to measure these dimensions, for example by applying predictive algorithms to existing secondary data like socioeconomic characteristics, socio-demographics, and condition of housing stock, would certainly help policy makers gain a better understanding of local communities. In their Policy & Internet article “Predicting Sense of Community and Participation by Applying Machine Learning to Open Government Data”, Alessandro Piscopo, Ronald Siebes, and Lynda Hardman employ a machine-learning technique (“Random Forests”) to evaluate an estimate of community capacity derived from open government data, and determine the most important predictive variables. The resulting models were found to be more accurate than those based on traditional statistics, demonstrating the feasibility of the Random Forests technique for this purpose—being accurate, able to deal with small data sets and nonlinear data, and providing information about how each variable in the dataset contributes to predictive accuracy. We caught up with the authors to discuss their findings: Ed.: Just briefly: how did you do the study? Were you essentially trying to find which combinations of variables available in Open Government Data predicted “sense of community and participation” as already measured by surveys? Authors: Our research stemmed from an observation of the measures of social…