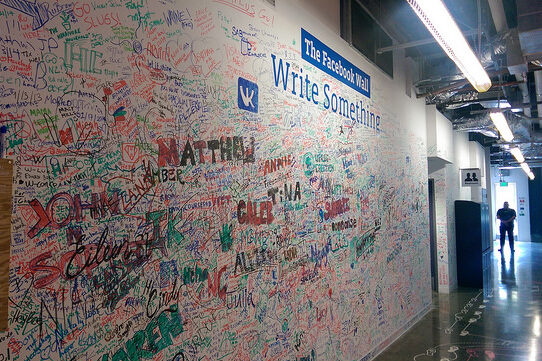
The Facebook Wall, by René C. Nielsen (Flickr).
A central ideal of democracy is that political discourse should allow a fair and critical exchange of ideas and values. But political discourse is unavoidably mediated by the mechanisms and technologies we use to communicate and receive information—and content personalisation systems (think search engines, social media feeds and targeted advertising), and the algorithms they rely upon, create a new type of curated media that can undermine the fairness and quality of political discourse. A new article by Brent Mittlestadt explores the challenges of enforcing a political right to transparency in content personalisation systems. Firstly, he explains the value of transparency to political discourse and suggests how content personalisation systems undermine open exchange of ideas and evidence among participants: at a minimum, personalisation systems can undermine political discourse by curbing the diversity of ideas that participants encounter. Second, he explores work on the detection of discrimination in algorithmic decision making, including techniques of algorithmic auditing that service providers can employ to detect political bias. Third, he identifies several factors that inhibit auditing and thus indicate reasonable limitations on the ethical duties incurred by service providers—content personalisation systems can function opaquely and be resistant to auditing because of poor accessibility and interpretability of decision-making frameworks. Finally, Brent concludes with reflections on the need for regulation of content personalisation systems. He notes that no matter how auditing is pursued, standards to detect evidence of political bias in personalised content are urgently required. Methods are needed to routinely and consistently assign political value labels to content delivered by personalisation systems. This is perhaps the most pressing area for future work—to develop practical methods for algorithmic auditing. The right to transparency in political discourse may seem unusual and farfetched. However, standards already set by the U.S. Federal Communication Commission’s fairness doctrine—no longer in force—and the British Broadcasting Corporation’s fairness principle both demonstrate the importance of the idealised version of political discourse described here. Both precedents…