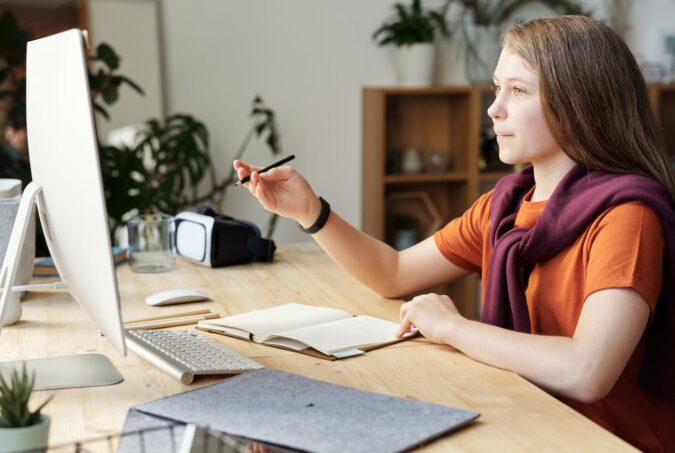
The Internet has provided the social, individual, and technological circumstances needed for child pornography to flourish. Sex offenders have been able to utilise the Internet for dissemination of child pornographic content, for social networking with other pedophiles through chatrooms and newsgroups, and for sexual communication with children. A 2009 estimate by the United Nations estimates that there are more than four million websites containing child pornography, with 35 percent of them depicting serious sexual assault [1]. Even if this report or others exaggerate the true prevalence of those websites by a wide margin, the fact of the matter is that those websites are pervasive on the world wide web.
Despite large investments of law enforcement resources, online child exploitation is nowhere near under control, and while there are numerous technological products to aid in finding child pornography online, they still require substantial human intervention. Despite this, steps can be taken to increase the automation process of these searches, to reduce the amount of content police officers have to examine, and increase the time they can spend on investigating individuals.
While law enforcement agencies will aim for maximum disruption of online child exploitation networks by targeting the most connected players, there is a general lack of research on the structural nature of these networks; something we aimed to address in our study, by developing a method to extract child exploitation networks, map their structure, and analyse their content. Our custom-written Child Exploitation Network Extractor (CENE) automatically crawls the Web from a user-specified seed page, collecting information about the pages it visits by recursively following the links out of the page; the result of the crawl is a network structure containing information about the content of the websites, and the linkages between them [2].
We chose ten websites as starting points for the crawls; four were selected from a list of known child pornography websites while the other six were selected and verified through Google searches using child pornography search terms. To guide the network extraction process we defined a set of 63 keywords, which included words commonly used by the Royal Canadian Mounted Police to find illegal content; most of them code words used by pedophiles. Websites included in the analysis had to contain at least seven of the 63 unique keywords, on a given web page; manual verification showed us that seven keywords distinguished well between child exploitation web pages and regular web pages. Ten sports networks were analysed as a control.
The web crawler was found to be able to properly identify child exploitation websites, with a clear difference found in the hardcore content hosted by child exploitation and non-child exploitation websites. Our results further suggest that a ‘network capital’ measure—which takes into account network connectivity, as well as severity of content—could aid in identifying the key players within online child exploitation networks. These websites are the main concern of law enforcement agencies, making the web crawler a time saving tool in target prioritisation exercises. Interestingly, while one might assume that website owners would find ways to avoid detection by a web crawler of the type we have used, these websites—despite the fact that much of the content is illegal—turned out to be easy to find. This fits with previous research that has found that only 20-25 percent of online child pornography arrestees used sophisticated tools for hiding illegal content [3,4].
As mentioned earlier, the huge amount of content found on the Internet means that the likelihood of eradicating the problem of online child exploitation is nil. As the decentralised nature of the Internet makes combating child exploitation difficult, it becomes more important to introduce new methods to address this. Social network analysis measurements, in general, can be of great assistance to law enforcement investigating all forms of online crime—including online child exploitation. By creating a web crawler that reduces the amount of hours officers need to spend examining possible child pornography websites, and determining whom to target, we believe that we have touched on a method to maximise the current efforts by law enforcement. An automated process has the added benefit of aiding to keep officers in the department longer, as they would not be subjugated to as much traumatic content.
There are still areas for further research; the first step being to further refine the web crawler. Despite being a considerable improvement over a manual analysis of 300,000 web pages, it could be improved to allow for efficient analysis of larger networks, bringing us closer to the true size of the full online child exploitation network, but also, we expect, to some of the more hidden (e.g., password/membership protected) websites. This does not negate the value of researching publicly accessible websites, given that they may be used as starting locations for most individuals.
Much of the law enforcement to date has focused on investigating images, with the primary reason being that databases of hash values (used to authenticate the content) exists for images, and not for videos. Our web crawler did not distinguish between the image content, but utilising known hash values would help improve the validity of our severity measurement. Although it would be naïve to suggest that online child exploitation can be completely eradicated, the sorts of social network analysis methods described in our study provide a means of understanding the structure (and therefore key vulnerabilities) of online networks; in turn, greatly improving the effectiveness of law enforcement.
[1] Engeler, E. 2009. September 16. UN Expert: Child Porn on Internet Increases. The Associated Press.
[2] Westlake, B.G., Bouchard, M., and Frank, R. 2012. Finding the Key Players in Online Child Exploitation Networks. Policy and Internet 3 (2).
[3] Carr, J. 2004. Child Abuse, Child Pornography and the Internet. London: NCH.
[4] Wolak, J., D. Finkelhor, and K.J. Mitchell. 2005. “Child Pornography Possessors Arrested in Internet-Related Crimes: Findings from the National Juvenile Online Victimisation Study (NCMEC 06–05–023).” Alexandria, VA: National Center for Missing and Exploited Children.
Read the full paper: Westlake, B.G., Bouchard, M., and Frank, R. 2012. Finding the Key Players in Online Child Exploitation Networks. Policy and Internet 3 (2).
2 Comments
Comments are closed.
This is very interesting stuff both from the aspect of being an IT professional and from being a local Councillor interested in social problems. It’s good to see the work that is being done to combat this particularly unpleasant problem.
The one concern I have is that if the crawlers mentioned in the article are visiting these illegal sites, doesn’t that increase their hit rate and encourage more offenders to either contribute to existing sites or start up their own? Both activities that would risk more child exploitation happening.
Tony, thanks for your comment. On the hit rate, you are correct, it does increase it, but only minimally. Most commonly, the web-crawler visits the website once. We may visit another time for validation purposes. These few visits are unlikely to increase their stats significantly. But this is something to keep in mind for future research on these issues.