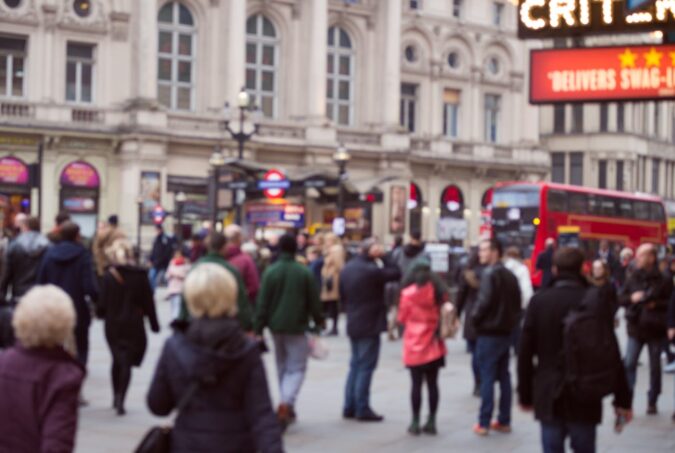
One of the big social science questions is how our individual actions aggregate into collective patterns of behaviour (think crowds, riots, and revolutions). This question has so far been difficult to tackle due to a lack of appropriate data, and the complexity of the relationship between the individual and the collective. Digital trails are allowing Social Scientists to understand this relationship better.
Small changes in individual actions can have large effects at the aggregate level; this opens up the potential for drawing incorrect conclusions about generative mechanisms when only aggregated patterns are analysed, as Schelling aimed to show in his classic example of racial segregation.
Part of the reason why it has been so difficult to explore this connection between the individual and the collective—and the unintended consequences that arise from that connection—is lack of proper empirical data, particularly around the structure of interdependence that links individual actions. This relational information is what digital data is now providing; however, they present some new challenges to the social scientist, particularly those who are used to working with smaller, cross-sectional datasets. Suddenly, we can track and analyse the interactions of thousands (if not millions) of people with a time resolution that can go down to the second. The question is how to best aggregate that data and deal with the time dimension.
Interactions take place in continuous time; however, most digital interactions are recorded as events (i.e. sending or receiving messages), and different network structures emerge when those events are aggregated according to different windows (i.e. days, weeks, months). We still don’t have systematic knowledge on how transforming continuous data into discrete observation windows affects the networks of interaction we analyse. Reconstructing interpersonal networks (particularly longitudinal network data) used to be extremely time consuming and difficult; now it is relatively easy to obtain that sort of network data, but modelling and analysing them is still a challenge.
Another problem faced by social scientists using digital data is that most social networks are multiplex in nature, that is, we belong to many different networks that interact and affect each other by means of feedback effects: How do all these different network structures co-evolve? If we only focus on one network, such as Twitter, we lose information about how activity in other networks (like Facebook, or email, or offline communication) is related to changes in the network we observe. In our study on the Spanish protests, we only track part of the relevant activity: we have a good idea of what was happening on Twitter, but there were obviously lots of other communication networks simultaneously having an influence on people’s behaviour. And while it is exciting as a social scientist to be able to access and analyse huge quantities of detailed data about social movements as they happen, the Twitter network only provides part of the picture.
Finally, when analysing the cascading effects of individual actions there is also the challenge of separating out the effects of social influence and self-selection. Digital data allow us to follow cascading behaviour with better time resolution, but the observational data usually does not help discriminate if people behave similarly because they influence and follow each other or because they share similar attributes and motivations. Social scientists need to find ways of controlling for this self-selection in online networks; although digital data often lacks the demographic information that allows applying this control, digital technologies are also helping researchers conduct experiments that help them pin down the effects of social influence.
Digital data is allowing social scientists pose questions that couldn’t be answered before. However, there are many methodological challenges that need solving. This talk considers a few, emphasising that strong theoretical motivations should still direct the questions we pose to digital data.
Further reading:
Gonzalez-Bailon, S., Borge-Holthoefer, J. and Moreno, Y. (2013) Broadcasters and Hidden Influentials in Online Protest Diffusion. American Behavioural Scientist (forthcoming).
Gonzalez-Bailon, S., Wang, N., Rivero, A., Borge-Holthoefer, J., and Moreno, Y. (2012) Assessing the Bias in Communication Networks Sampled from Twitter. Working Paper.
Gonzalez-Bailon, S., Borge-Holthoefer, J., Rivero, A. and Moreno, Y. (2011) The Dynamics of Protest Recruitment Through an Online Network. Scientific Reports 1, 197. DOI: 10.1038/srep00197
González-Bailón, S., Kaltenbrunner, A. and Banchs, R.E. (2010) The Structure of Political Discussion Networks: A Model for the Analysis of Online Deliberation. Journal of Information Technology 25 (2) 230-243.